Built with BigQuery: BigCommerce teams up with Tech Partners to make gathering, analyzing and acting on retail data easy
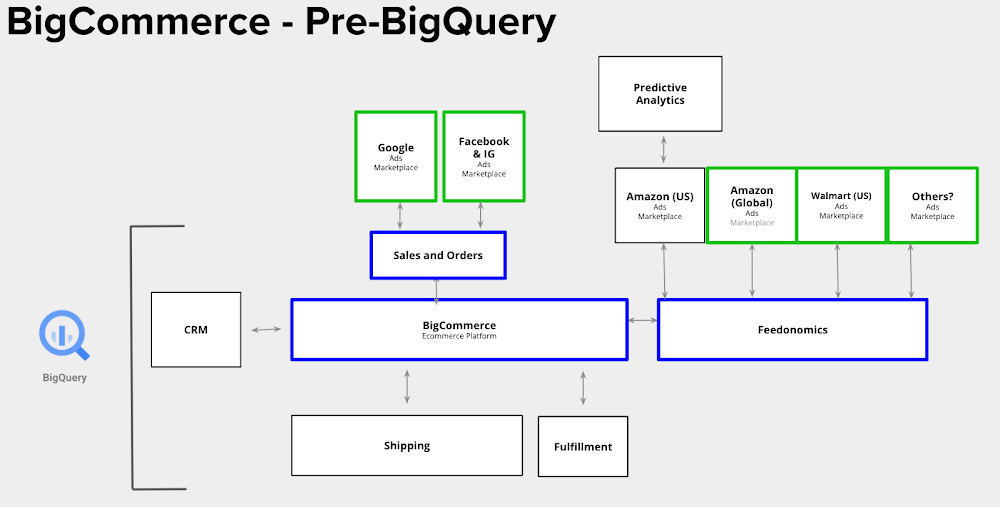
Data sets are, undoubtedly, one of the most valuable resources of the 21st century. Fortune 500 companies and small businesses alike can leverage data to influence make-or-break decisions across their online network. If you have a question, the answer probably lies somewhere within your data.
But gathering and interpreting that user data, then using it to influence business decisions, is often easier said than done. With a myriad of data collection software and even more aggregation tools, it can be challenging for businesses to make decisions based on the data at their disposal.
That’s why BigCommerce has teamed up with Google Cloud to natively integrate BigQuery with our Open-SaaS ecommerce platform. This integration will give merchants using our platform a powerful new tool to gather, analyze and act on their valuable data from one, convenient location.
Use-cases: Challenges and problems solved
It’s not the data themselves that are challenging; it’s the sheer volume. Statista estimates that, by 2025, there will be over 181 zettabytes of data created, captured, copied and consumed online. That’s a lot of data. And when all of that data is gathered using different sources or tools, creating actionable insights like where or how to advertise, messaging, product goals, and more, can cause huge challenges.
Enterprise merchants can often see tens of thousands of visitors a day to their site. Ads can cross the screens of hundreds of thousands of potential customers using a myriad of channels. Using that data to make informed business decisions is imperative for merchant success.
Many merchants struggle with this data analysis because of a lack of a single source of truth. Different teams or departments may use different tools to aggregate data, and even then those data are often siloed, which creates a non-cohesive approach to sales and overall ecommerce strategy.
BigQuery for BigCommerce enables merchants to consolidate all their data into a single source of truth and utilize structured query language (SQL) and Google Looker Studio to analyze and visualize their data. This empowers merchants to make strategic business decisions based on real-world insights.
Solution
Let’s explore an example of a merchant both before and after using Google BigQuery to aggregate and analyze their data.
This merchant sells both business-to-business (B2B) and direct-to-consumer (DTC). While they have an ecommerce website, they also use a number of other sales channels to maximize growth, including Facebook, Instagram, Amazon and Google. In addition to selling, they also advertise on these platforms in order to expand their reach and attract more customers.
Through their BigCommerce backend, they are able to integrate with a wide variety of services, either natively or through a third–party. But all of the data gathered from these services — advertising, omnichannel selling, their ecommerce store, etc. — is siloed in its own platform. Marketing teams keep ad data in its own program; ecommerce teams keep sales data in their platform of choice. Even individual teams within departments can use different tools to analyze data.
How does this impact the merchant? It keeps them from analyzing and acting on the data in a timely and effective manner. For example, let’s say this particular merchant is A/B testing a call to action across their ads. They may be able to see which ads received more clicks, but attributing those clicks to sales on their backend would be extremely challenging because of a lack of cohesive data. It would take too long to perform any meaningful analysis, and by the time one was finished, the data may be irrelevant.
This is just one of many examples as to how siloing data can negatively impact a merchant’s bottom line.
Now let’s look at the example architecture with BigQuery integrated into the data stream.
The merchant is still advertising and selling across multiple platforms. They are still gathering massive amounts of data through testing, sales and other means. Following this new approach, instead of all of that data staying in one location, it can now move freely between the BigCommerce backend and BigQuery.
The BigQuery integration with BigCommerce enables merchants to extract their store’s data and analyze it in real-time, providing valuable insights into their business performance. BigCommerce sends data to BigQuery via a REST API, which includes information on sales, customers, products, and more. BigQuery then stores and processes the data, allowing merchants to run complex queries and generate reports. The integration is facilitated by a data transfer service that ensures the data is securely transferred and automatically refreshed on a scheduled basis. The different platforms communicate through API requests and responses, ensuring seamless and efficient data transfer between BigCommerce and BigQuery.
BigQuery integrated with BigCommerce provides faster and more efficient data processing and analysis. It also offers increased reliability and security of data storage and processing through Google’s cloud infrastructure. This integration can lead to cost savings for merchants by avoiding investments in hardware and software, and it has a pay-per-query pricing model. Merchants can make real-time decisions based on current insights, instead of outdated data analysis.
This is a significant improvement to the previous model. Before, by the time these data were gathered and analyzed, no significant action could be taken because the data would be outdated.
Now, by integrating with BigQuery, the merchant has the ability to run their collected data through a variety of reporting and business intelligence platforms. Using these tools, merchants can aggregate, analyze and gain actionable insights on their valuable data quickly and efficiently. That means the potential for more sales and more time to grow your business.
Better together with Google Cloud
BigCommerce allows merchants to connect to some of the most powerful tools in tech. Using our native integrations with Google Cloud tools like BigQuery and Looker Studio, merchants can now easily gather, analyze and act on data from sales channels, advertising platforms and more, all without the need for massive development schedules or internal budgets. This partnership is just another way that BigCommerce is working to become the most modern enterprise ecommerce platform.
Learn more about BigCommerce’s Big Open Data Solutions here.
The Built with BigQuery advantage for ISVs
Google is helping tech companies like BigCommerce build innovative applications on Google’s data cloud with simplified access to technology, helpful and dedicated engineering support, and joint go-to-market programs through the Built with BigQuery initiative, launched in April ‘22 as part of the Google Data Cloud Summit. Participating companies can:
Get started fast with a Google-funded, pre-configured sandbox.
Accelerate product design and architecture through access to designated experts from the ISV Center of Excellence who can provide insight into key use cases, architectural patterns, and best practices.
Amplify success with joint marketing programs to drive awareness, generate demand, and increase adoption.
BigQuery gives ISVs the advantage of a powerful, highly scalable data warehouse that’s integrated with Google Cloud’s open, secure, sustainable platform. And with a huge partner ecosystem and support for multi-cloud, open source tools and APIs, Google provides technology companies the portability and extensibility they need to avoid data lock-in.
Click here to learn more about Built with BigQuery.
We thank the Google Cloud and BigCommerce team members who co-authored the blog: BigCommerce: Liz O’Neill, Associate Product Marketing Manager; Reed Hartman, Content Marketing Manager. Google: Sujit Khasnis, Solutions Architect
Source : Data Analytics Read More